The first part of a two-part blog series highlighted key takeaways from the 23rd RDA Plenary Meeting in San José, Costa Rica, presenting the AI/ML and interoperability topics that are relevant to FAIRLYZ. This second part will present and discuss current efforts to develop practical omics data curation workflows that include data annotation, curation, and versioning which are strong focus points in FAIRLYZ.
Data Curation
The session titled “Effectively Utilizing Research Tools in Curation Processes” was organized by the Research Tools and Data Curation Workflows IG and focused on the challenges and opportunities of integrating research tools and data curation practices. One use case provided by Datacite describes how to use persistent identifiers (PIDs) in research tools to support FAIR workflows. Another use case discussed experiences using the electronic lab notebook Rspace and implementing end-to-end FAIR workflow support. The goal is to implement research workflows that are facilitated by tool interoperability. One problem is that many people are involved in designing or deciding on the interoperability solution but roles and responsibilities are unclear: PID organizations, PID integrators, developers of research tool(s), institution leaders, communities of practice, and researchers. The integration of data curation into FAIR Workflows, shown below, remains an ongoing challenge.
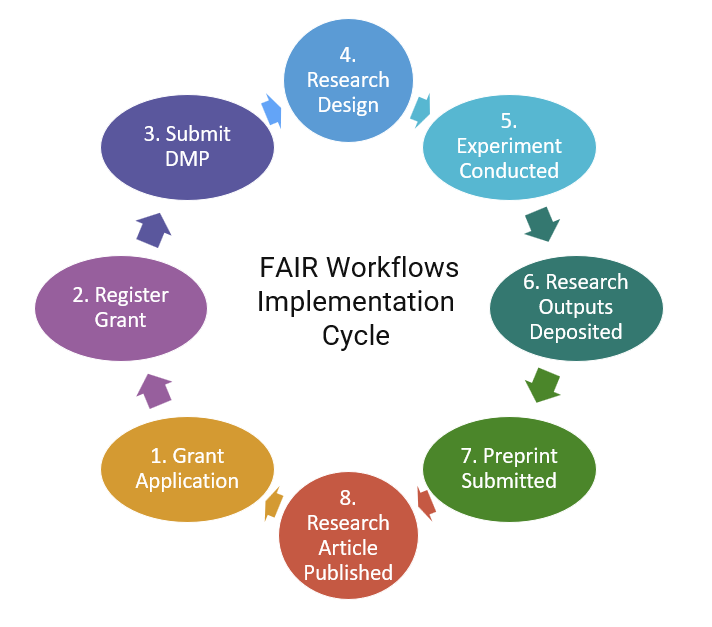
Evaluating Multi-Omics Data Standards
The session titled “Evaluating Multi-Omics Data Standard Integration Challenges and Building Solutions” organized by the Multi-omics Metadata Standards Integration (MOMSI) WG featured three compelling use cases on the complexities of integrating multi-omics data standards:
- Challenges faced by ProteomeXchange in the adoption of SDRF-Proteomics led to the development of lesSDRF, a user-friendly web app for generating SDRF-Proteomics metadata files. The MIxS standard was integrated into SDRF-Proteomics to facilitate the capture of metadata for metaproteomics studies.
- MARS, a Multi-omics Adapter for Repository Submissions uses ISA-JSON to exchange information between data producers and data repositories. MARS submits to various European EMBL-EBI repositories such as: European Nucleotide Archive (ENA), ArrayExpress, PRIDE, MetaboLights, EVA, and Mgnify. MARS is being adopted by the ELIXIR Data platform. A question was asked about the ability of MARS to submit to NIH repositories and the answer is that it is possible but requires coordination.
- A third presentation described the modeling of mass spectrometry metadata in the National Microbiome Data Collaborative (NMDC) to enable multi-omics data reusability. The NMDC Metadata, originally designed for Metagenomic and Metatranscriptomic data, was refactored for metabolomics and lipidomics metadata, facing several challenges due to very different metadata between these two types of ‘omics data.
Active Data Management Plans
The session “Active Data Management Plans: What are the actions that we need to realize them?” organized by Active Data Management Plans IG and DMP Common Standards WG presented implementations of the Salzburg Manifesto: “Ten principles for machine-actionable data management plans” (maDMPs). These principles are:
- Integrate DMPs with the workflows of all stakeholders in the research data ecosystem
- Allow automated systems to act on behalf of stakeholders
- Make policies (also) for machines, not just for people
- Describe—For both machines and humans—The components of the data management ecosystem
- Use PIDs and controlled vocabularies
- Follow a common data model for maDMPs
- Make DMPs available for human and machine consumption
- Support data management evaluation and monitoring
- Make DMPs updatable, living, versioned documents
- Make DMPs publicly available
The session also discussed the integration of RDA DMP Common Standards with other initiatives, such as the European Open Science Cloud (EOSC) and Datacite and necessary steps towards FAIRness, interconnectivity and machine actionability across research phases.
Example of Tools or organizations that have adopted the principles are shown below.
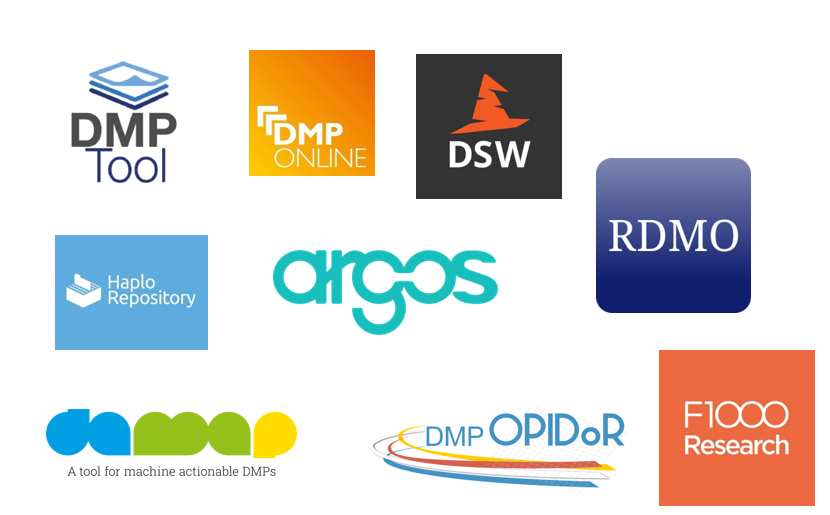
The Machine Actionable Plans (MAP) aims to improve data management and sharing by enhancing the DMP Tool and persistent identifier registries. However, due to the limitations of the current technology, a rebuild is necessary to achieve these goals as described in Announcing the DMP Tool Rebuild. Some of the areas that have been prioritized include:
- Additional API functionality for unpublished or in-progress DMPs
- Ability to upload and register existing DMPs
- Improved account management, e.g. adding secondary emails
- Increased flexibility in creating and copying templates
- Finding and connecting DMPs to published research outputs, e.g. datasets
- Improved notification, comment, and feedback systems
Ostrail which co-develops and tests fit-for-purpose pathways and solutions presented their planning-tracking-assessing (PTA) framework for FAIRness and machine actionability across domains and national settings. They are working with 15 national, 8 thematic, 1 paneuropean initiatives in Europe to implement interoperability frameworks for more than 70 tools.
Other topics of interests were how to maintain the RDA DMP Standards in Github to implement small changes and resolve legacy issues, and how to implement common APIs for DMP Platforms (Zoubek, F. 2023).
Data Versioning
The session titled “What do we actually mean when we talk about data versioning?” was presented by the RDA Data Versioning IG. The session discussed actionable guidelines on data versioning practices using persistent identifiers (PIDs) based on 54 use cases collected globally. The aspects relevant to FAIRLYZ were related to data re-publication, mirroring and re-using data. The guidelines used concepts from software versioning as well as the Functional Requirements for Bibliographic Records (FRBR) as a conceptual framework. The 3 critical drivers for data versioning are (1) authorship, (2) reproducibility, and (3) State of data curation or quality. Distinctions are made between collections of data items and time series data. Questions that are relevant to the FAIRLYZ registry are:
- What constitutes a new release of a dataset, and how should it be identified?
- Should metadata be versioned?
- How should provenance be expressed in a versioning history?
- How to differentiate between major and minor changes
- When are results of analyses considered to be “data”?
Among the relevant recommendations are:
- Collections of data have one PID, but individual items may also have PIDs
- Time series in which data are only appended can be identified by a PID
- The mirrored or re-published data should always refer to the original source (the authoritative version which is called “Original data” in FAIRLYZ)
- If both the data and the metadata have become unavailable, their PID should resolve to a tombstone page (This created conflicting responses as some suggested metadata should always be preserved)
- Clearly communicate to users which identifier is used for the object/data described by the metadata and which identifier refers to the metadata record
A key aspect missing from the discussions was the interconnectedness of data curation, multi-omics standards, active data management plans, and data versioning. These elements belong to a cohesive omics data curation workflow, ensuring data quality and reproducibility.